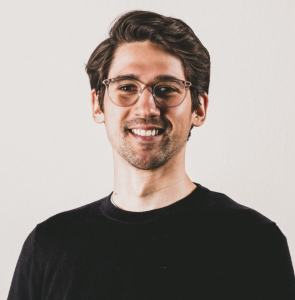
Transcloud
April 8, 2025
April 8, 2025
Revolutionizing Machine Learning Operations with GCP
As AI revolutionizes industries, businesses are racing to harness its potential for faster decision-making, intelligent automation, and competitive advantage. However, the journey from raw data to production-ready ML models is filled with challenges—inconsistent data quality, complex pipelines, and deployment inefficiencies can hinder success.
This is where MLOps (Machine Learning Operations) comes in, merging ML engineering with DevOps best practices to streamline workflows, automate processes, and enable continuous model improvement. Among cloud providers, Google Cloud Platform (GCP) stands out as a powerful, scalable, and AI-optimized ecosystem that simplifies the entire ML lifecycle.
At Transcloud, we specialize in leveraging MLOps on GCP to help organizations accelerate AI adoption, optimize ML workflows, and drive real business impact. In this blog, we’ll explore how GCP’s advanced MLOps capabilities are transforming machine learning deployment, scalability, and operational efficiency—ensuring businesses stay ahead in an increasingly AI-driven world.
Every successful ML project relies on a well-structured workflow—a systematic process that transforms raw data into a deployable and maintainable model. Without clear workflows, projects can become chaotic, leading to inefficiencies and unreliable models.
A streamlined workflow enhances every stage of an ML project. Here’s how:
Despite the importance of structured workflows, many ML projects face significant roadblocks that slow progress and reduce effectiveness.
Organizations often struggle with the following ML workflow challenges:
Addressing these challenges requires a robust MLOps framework that automates workflows and ensures efficiency. This is where Google Cloud’s MLOps capabilities make a difference.
MLOps integrates ML engineering with DevOps principles, ensuring that ML models are not just built, but also deployed and maintained efficiently. It focuses on automation, collaboration, and continuous improvement, making ML workflows more agile and effective.
By following these principles, organizations can make ML models more reliable, scalable, and adaptable to changing business needs.
At the core of every ML workflow is data engineering—the foundation that ensures smooth data processing and quality control. Data engineering enables:
Without strong data engineering practices, even the most sophisticated ML models can fail to deliver value. GCP provides a suite of tools that streamline data engineering as part of its MLOps offering.
Vertex AI in Google Cloud offers a comprehensive ecosystem designed to simplify every stage of the ML lifecycle.
Vertex AI is an all-in-one platform that integrates data preparation, model training, deployment, and monitoring. It allows teams to:
A powerful framework for building and deploying ML models, TensorFlow seamlessly integrates with GCP to provide scalable machine learning solutions.
A collaborative repository that enables teams to share and discover pre-trained ML models, pipelines, and components, reducing development time.
By leveraging these tools, organizations can develop and manage ML solutions faster and more effectively.
One of the biggest advantages of GCP is automated model training. With Vertex AI’s AutoML, developers can build high-performing models without requiring deep ML expertise. Key features include:
Automation not only saves time but also ensures that models perform at their best without manual intervention.
Deploying ML models is often a challenging task, but GCP simplifies it with:
By automating deployment and monitoring, organizations can maintain high-performing models without constant manual oversight.
MLOps on Google Cloud is not just about simplifying workflows—it’s about enabling businesses to unlock the full potential of AI. Key benefits include:
By embracing MLOps on GCP, organizations can stay ahead of the competition and drive long-term innovation.
The future of AI-driven business success lies in scalable, automated, and continuously improving ML workflows. MLOps on Google Cloud enables organizations to break down silos, reduce inefficiencies, and deploy high-performing ML models with confidence. With Vertex AI, AutoML, and seamless CI/CD pipelines, businesses can accelerate decision-making, optimize operations, and stay ahead in an increasingly data-driven landscape.
At Transcloud, we specialize in designing tailored MLOps solutions on GCP, ensuring organizations maximize efficiency, minimize complexity, and scale AI adoption with ease. Whether you’re a startup exploring AI possibilities or an enterprise deploying ML at scale, our expertise in cloud-based ML solutions ensures that your AI investments drive measurable business impact.
Now is the time to embrace MLOps with Transcloud—because the future of AI is not just about building models; it’s about making them work smarter, faster, and better for your business.